Robotic Skills November 2016
Want more free featured content?
Subscribe to Insights in Brief
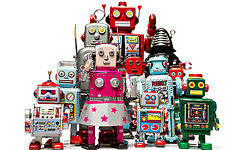
Researchers are continually developing robots that possess new skills and abilities that enable them to complete specific complex tasks autonomously. Examples of such capabilities are manifold. A group from the University of Tokyo (Tokyo, Japan) has developed a robot capable of playing the game rock-paper-scissors with a human and winning every time. The robot uses a high-speed camera and image-processing software to determine the shape the opponent is making with his or her hand and then quickly articulates its hand into the winning shape. Researchers at the Georgia Institute of Technology (Atlanta, Georgia) have developed a wearable robotic arm to assist musicians in playing a drum set. The arm analyzes the drummer's movements, chooses an appropriate drum, and plays a rhythm in time with the drummer. Researchers from the Children's National Medical Center (Washington, DC) have developed a surgical robot capable of autonomously suturing incisions in the intestinal tissue of living pigs. The robot uses a 3D camera and fluorescent tags to track the soft tissue as it sutures an incision closed. Moley Robotics (London, England) is developing an automated-kitchen system that features two suspended robotic arms that mimic the movements of professional chefs. The company uses 3D-motion-capure technology to film a human chef preparing a dish, and the robotic arms learn to make the dish by replicating the chef's movements.
To date, efforts to integrate multiple skills into a single robot have seen only moderate success.
These examples highlight continuing advances in robotics research, but each project demonstrates a robot with only one very specific application. Although these projects prove that robots have many potential applications, they do not necessarily advance overall robotics research. Many potential commercial opportunities and applications depend on the development of a general-purpose robot with a broad skill set. To date, efforts to integrate multiple skills into a single robot have seen only moderate success. In June 2015, a number of the world's most advanced robotic systems competed at the finals of the Robotics Challenge hosted by the US Department of Defense's (Arlington County, Virginia) Defense Advanced Research Projects Agency (DARPA; Arlington, Virginia). For the Robotics Challenge, teams of researchers attempted to develop humanoid robots capable of completing eight tasks common in search-and-rescue operations, including walking over uneven terrain, walking up stairs, opening doors, turning valves, and operating power tools. Only 3 of the 23 teams that participated in the finals were able to complete all eight tasks. Many of the robots fell and suffered damages severe enough to prevent them from continuing through the course. After the event, DARPA posted a video that shows some of the more spectacular robot failures (www.youtube.com/watch?v=7A_QPGcjrh0). The competition demonstrated the difficulty of integrating multiple skills into a single general-purpose robot and highlighted the need for a substantial amount of additional research. For instance, SRI International (Menlo Park, California) is working with Yamaha Motor Company (Iwata, Japan) to develop a robot capable of autonomously riding a motorcycle. Rather than altering a motorcycle to make it suitable for use by a robot, the team is designing the robot to ride an unmodified motorcycle in the same way humans do. General-purpose robots will likely need to possess a range of basic skills, including voice recognition, object recognition, object grasping and manipulation, localization, and mobility control (either through wheels or bipedal walking).
In addition to possessing a range of basic skills, robots will need a method to learn new skills. One approach is to enable robots to gain new skills through cloud-based knowledge sharing. RoboHow (https://robohow.eu) is a four-year European research project that is building on the progress of the RoboEarth (http://roboearth.ethz.ch) research project. RoboHow's goal is to develop a platform that uses web resources and experience-based learning to enable robots to acquire new skills automatically. Researchers have used the platform to teach robots how to flip pancakes and roll pizza dough. A team comprising researchers from Stanford University (Stanford, California), Cornell University (Ithaca, New York), and other institutions is working on the Robo Brain (http://robobrain.me) cloud-based computational system. The system learns from online resources, computer simulations, and robotic trials to develop a single comprehensive knowledge base in the cloud. The team hopes that the system will enable robots to learn collectively and share maps, images, object data, and other information. A previous Scan™ article, In Deep Learning, Quantity Matters, highlights examples of efforts to use large data sets to train deep-learning algorithms.
Other researchers are investigating how individual robots can learn new skills automatically. Researchers from the University of Washington (Seattle, Washington) are developing algorithms that enable a dexterous robotic hand they built to perform complex object manipulations. Machine-learning algorithms use data that a variety of sensors and motion-capture cameras collect as the robotic hand moves to learn and improve the hand's ability to manipulate objects. A team from Google DeepMind (Alphabet; Mountain View, California) is developing progressive neural networks—multiple neural networks that link to enable each network to contribute the specific function it has learned. This architecture enables a system to learn new tasks very quickly if portions of those tasks relate to functions the individual neural networks in the architecture had learned previously. Initially, the DeepMind team used progressive neural networks to train an artificial intelligence to play classic video games. Since then, the team has been working to use progressive neural networks to train a robotic arm to perform various tasks. The team used a computer simulation of a robotic arm to train a primary neural network and then added additional neural networks that made use of information from the primary network while learning to control a real robotic arm. This method enabled the team to reduce significantly the number of integrations necessary to train the arm to perform simple tasks. This approach could give individual robots a way to leverage previous learning to decrease the amount of training time necessary for them to learn a new skill.
As another previous Scan article, The (Ir)Rational Fear of Automation, highlights, many people are growing increasingly concerned that robots will replace a large number of human workers in the near future. Many of these fears stem from media coverage of rapid advances in artificial intelligence and deep-learning algorithms, but these advances have primarily been in software-only applications such as data analysis and image processing. Researchers are only beginning to apply such advances to solving complex problems in the field of robotics. Overall, progress in robotics has been limited, and developing a robot capable of automating a single task is a major accomplishment. Robots with a single skill will be able to automate only a specific portion of a human worker's job, and a general-purpose robot that can cook, clean, manipulate tools, and perform any physical task that humans can perform will remain elusive for years.