Big Data for Cybersecurity Update September 2017
Want more free featured content?
Subscribe to Insights in Brief
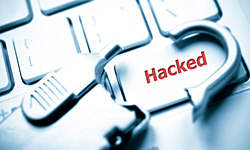
"Big Data for Cybersecurity" in Explorer's July 2016 Big Data Viewpoints outlines efforts to combat cyberthreats with big-data analytics and machine learning. Advocates of big-data approaches to cybersecurity hope that machine-learning software can monitor the volumes of data that information-technology systems produce to detect anomalies and to react to attacks in their early stages.
Investor interest in data-driven cybersecurity remains high. Cylance is still the best-funded start-up (its 2016 funding round raised $100 million on a valuation of $1 billion), though its competitors are catching up. Darktrace recently raised $75 million on a valuation of $825 million, and Awake Security recently emerged from stealth mode with $31 million of funding already in place. Darktrace uses unsupervised machine learning to build a model of normal system behavior and alerts analysts to anomalies. Awake Security uses predictive analytics to identify patterns across multiple security systems that may indicate corporate espionage, insider attacks, or data exfiltration. Dozens of other start-ups also claim to use machine learning to aid cyersecurity.
The open-source community is also active in data-driven cybersecurity. Apache Spot is an open-source initiative that aims to provide advanced analytics for enterprise-security data and create a standards-based platform for data integration. Spot contributors including Cloudera and Intel are working toward Spot's Version 1.0 release. Cloudera already offers its customers a cybersecurity solution based on its Enterprise Data Hub that will likely share many features with the forthcoming open-source software.
Implications
Although the supply side of the data-driven cybersecurity market is strong, the demand side is still developing. A survey of cybersecurity professionals by Enterprise Strategy Group (ESG) indicated that many professionals are unsure about the role of machine learning in security (only 30% of respondents said they were very knowledgeable about the area). In addition, some security analysts may be suspicious of start-ups that claim to automate some of their often complex and highly paid work—though in practice, current best-of-breed tools can only support, not automate, human security analysts.
Demand for data-driven cybersecurity is likely to catch up with supply as increasing numbers of success stories emerge, tools mature, and professionals become used to working with the technology. The ESG survey found that although only 12% of respondents said they had already deployed machine learning for cybersecurity extensively, almost all respondents (94%) had plans to make deployments in the future.
Impacts/Disruptions
Automating threat detection is likely the only plausible way for security professionals to keep pace with the quickly growing number of connected systems and devices that they need to protect. Manual approaches are simply unsustainable. But even with automation, network-connected systems and devices are unlikely ever to be completely secure. History suggests that advances in cybersecurity are matched by advances in cyberthreats. Perhaps future hackers will find ways of masking attacks within normal system behavior, or perhaps they will overwhelm systems with so many anomalies that systems will be unable to detect meaningful patterns. Like defenses today, future cybersecurity defenses will be imperfect. But they must at least keep pace with growing cyberthreats—perhaps a reason why investors are so optimistic that data-driven cybersecurity will generate returns.