Machine-Based HR June 2018
Want more free featured content?
Subscribe to Insights in Brief
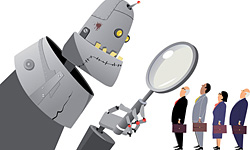
Artificial intelligence (AI) and machine learning (ML) have advanced rapidly during the past decade, and their use in human resources (HR) is a growth area. Although the use of AI and ML in HR provides many benefits for employers and employees alike, some problematic aspects deserve attention. In 2016, Scan™ Signal of Change Algorithmic HR mentions that the use of AI and ML in HR applications is becoming increasingly common. The Scan Signal of Change HR, AI, and EI from 2017 cautions that operational excellence will depend on understanding the trade-offs between AI and emotional intelligence (EI).
Algorithmic advances make resisting the use of machine-based decision making difficult for managers. Algorithmic HR promises not only to improve on operational excellence—by, for instance, finding the right people for various positions—but also to circumvent human bias and prejudice. Josh Bersin, founder and principal of HR consultancy Bersin by Deloitte (Deloitte Touche Tohmatsu; London, England), argues that AI-based tools are transforming HR processes in significant ways. He highlights that conversational interfaces facilitate interactions between humans and information-technology (IT) systems and that ML enables software to perform advanced analyses of HR information to provide detailed recommendations and even make decisions. Bersin also sees increasing use of predictive models to identify problem areas of performance or even criminal behavior in advance. According to Bersin, "Since most of the non-transactional parts of HR are based on judgment—virtually every management and people decision is based on imperfect data—I believe AI in HR has the potential to improve and automate many of the ad hoc decisions we make on a daily basis" ("How will AI in HR be a game-changer?" SearchHRSoftware, December 2017; online).
The introduction of AI systems to HR processes will have far-reaching implications for employees, starting with effects on their access to employment opportunities. Companies are experimenting with the use of machine-based analytics to identify the best job candidates for a given position. Individuals face such scrutiny not only when seeking new employment with a company but also when trying to advance within the company that already employs them. AI systems see use to evaluate a wide range of candidates' capabilities and dispositions by analyzing candidates' microgestures and word choices and even the tone of their social-media posts. An entire ecosystem of software providers is catering to every aspect of HR selection processes. For example, Entelo (San Francisco, California) provides software that enables recruiters to search the internet and social-media platforms for information about candidates, including whether a candidate is likely to switch jobs. Talent Sonar (San Francisco, California) offers ML algorithms that compose job descriptions that help companies attract the best candidates and circumvent various unconscious biases that managers in charge of hiring may have. HireVue (South Jordan, Utah) uses voice-recognition software, facial-recognition software, and a ranking algorithm to analyze candidates' video interviews and identify which candidates are likeliest to meet the needs of hiring companies. Even Google (Alphabet; Mountain View, California) has started offering algorithmic solutions for HR applications. For instance, Cloud Job Discovery "provides plug and play access to Google's search and machine learning capabilities, enabling the entire recruiting ecosystem...to improve job site engagement and candidate conversion" (https://cloud.google.com/job-discovery). Josh Bersin claims that roughly 75 start-ups are now offering their services and applications to the HR-assessment market, which is worth about $100 billion.
HR practitioners see the most beneficial use of such software-based assessment tools at the beginning of selection processes. At this stage of hiring, such tools can reduce the applicant pool to a manageable size, enabling HR practitioners to conduct personal interviews with the remaining applicants—a process in which human judgment still plays the dominant role in decision making. Nevertheless, an applicant sorted out by algorithmic criteria will never become a job candidate who can prove his or her value to interviewers. Because companies are increasingly using chat bots and online quizzes and games to prescreen applicants, job seekers face a very new hiring environment. "How to persuade a robot that you should get the job," a 3 March 2018 Guardian article, lists a number of challenges and hurdles that job seekers face. First, applicants now have to prepare not only for traditional steps in the job-application process but also for whichever types of algorithmic assessment tools hiring companies have chosen to use. Second, applicants and candidates may have no way to address a situation in which they believe an algorithm has discriminated against them because of an AI's built-in biases. Third, although many jobs (such as construction and retail jobs) do not require IT knowledge, assessment tools' moving online forces many applicants to face computer-related challenges that do not pertain to the job they are seeking—a particularly difficult problem for older applicants.
As data collection increases not only during job applications and interviews but also on a daily basis on the job, some observers worry about the implications the situation could have for privacy. Humanyze (Boston, Massachusetts) employs people analytics—its term for "using behavioral data to understand how people work and change how companies are managed" (www.humanyze.com). The company analyzes communication metadata such as email and phone-call time stamps, the number of chat messages employees sent, and meeting durations. This analysis reveals patterns within company teams, which can help a company better understand how employees share information so it can improve communication and collaboration. And Amazon.com (Seattle, Washington) recently received two patents for a wristband-based inventory-management system that can identify the location of warehouse employees and even track the movements of the employees' hands. Such data collection undoubtedly will enable companies to improve organizational processes and logistics; however, even the most well-intentioned systems use assumptions that can prove problematic under real-world work conditions, measure misleading metrics, and come to conclusions that could be counterproductive because important productivity aspects go unnoticed or prove immeasurable.
The increasing use of AI and ML in HR applications could improve productivity and efficiency and circumvent the biases of individuals (although programmers might inadvertently include biases in algorithms, thereby replacing one problem with another). The use of algorithms to assess individuals can have a dehumanizing effect, which should be a major concern for companies. Some people will appreciate a metrics-based evaluation, but others will find being judged by a machine an alienating experience. Whatever the case may be, transparency in the use of such systems and assessment criteria will be important to reducing conflict potential.